“Algorithm is arguably the single most important concept in our world.”
– Yuval Noah Harari, author of ‘Homo Deus: A History of Tomorrow’
23,012 Active Engagements

Introduction
As we navigate the frontier of technological innovation, the question of whether algorithms can take the reins of company leadership emerges with compelling urgency. This exploration delves into the transformative impact of algorithms on business decision-making, extending from enhancing operational efficiencies to potentially guiding strategic directions. The intriguing prospect of algorithms not just supporting but leading organizational efforts prompts a reevaluation of the traditional boundaries between human and algorithmic decision-making. This article seeks to uncover the reality of a future where algorithms could drive companies toward unparalleled growth and competitive advantage, marking a pivotal shift in the corporate world’s dynamics.
What is an Algorithm?
Algorithms, are like a set of instructions or a recipe that tells a computer how to solve a problem or perform a task. Just like a recipe guides you through the steps to make a dish, an algorithm guides the computer to achieve a specific outcome. They have increasingly become integral to the operation and success of companies, especially in the era of digital transformation. They are pivotal in a range of business functions, from optimizing processes and creating revenue streams to making more informed decisions based on data analysis.
Human Limitations: The Magical Number Seven, Plus or Minus Two
George A. Miller’s seminal paper highlights human brain limits in processing information, underscoring cognitive constraints in complex decision-making. Algorithms play a crucial role in bridging this gap. They enable businesses to manage, analyze, and glean insights from vast datasets, far beyond what the human mind can handle unaided. By effectively automating and optimizing data processing, algorithms are not just tools of efficiency; they are essential in overcoming the innate constraints of human cognition in the realm of complex decision-making combined with ever expanding massive data volumes. For context, small to medium-sized enterprises (SMEs) easily accumulate terabytes (TB) of data, while large corporations often deal with petabytes (PB) or even exabytes (EB) of data. This includes data from various sources such as customer information, transaction records, social media interactions, IoT device outputs, and operational data. Consider, the total global volume of data is set to explode over the coming years, amounting to an expected 181 zetabytes1 by 2025. (Statista, 2024)
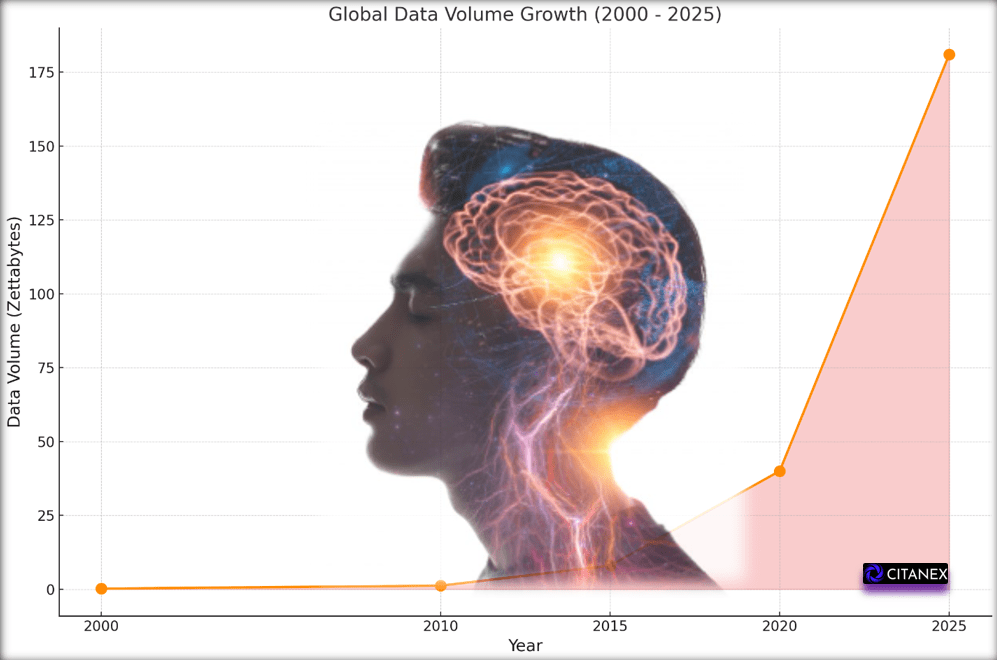
Moore’s Law and Singularity
Moore’s Law suggests that computer power doubles every two years, making technology faster and cheaper. The Singularity is a future moment when artificial intelligence (AI) might (and likely will) outsmart human intelligence, leading to massive changes, including how companies operate. These are key ideas in imagining the future of businesses run by technology. Together, these concepts hint at a world where companies could be managed by AI and algorithms, doing tasks and making decisions with speed and efficiency beyond what humans can achieve. This idea opens the door to a new era of business, where technology could lead to groundbreaking ways of working and innovating. Some experts predict that the Singularity will occur as early as 2029. In 2005, Ray Kurzweil2 predicted human-level AI around 2029, and the singularity in 2045. In a 2017 interview, Kurzweil reaffirmed his estimates. Although technological progress has been accelerating in most areas, it has been limited by the basic intelligence of the human brain, which has not, according to Paul R. Ehrlich3, changed significantly for millennia.
Singularity is the point at which “all the change in the last million years will be superseded by the change in the next five minutes.”
Kevin Kelly – Founding Executive Editor of Wired magazine
Applications of Algorithms in Business: Personalization and Engagement
The role of algorithms in business can be seen in various applications. For instance, they are used in areas such as e-commerce, where they help in offering personalized recommendations to customers based on their browsing and purchase history. This not only enhances the customer experience but also drives sales by highlighting products that are more likely to interest specific customer segments. Additionally, algorithms are employed in social media platforms to tailor content feeds according to user preferences, thereby increasing engagement and visibility for businesses using these platforms for marketing. (Salesforce, 2020)
Artificial Intelligence (AI): Machine Learning and Deep Learning
In more advanced applications, Machine Learning4 and Deep Learning5, subsets of Artificial Intelligence (AI), are leveraging algorithms to an even greater extent. These technologies enable computers to learn from data and improve over time, making decisions or predictions based on historical data. This has opened up new possibilities in areas like fraud detection, predictive maintenance6, and even in developing self-driving cars, as seen with companies like Tesla.
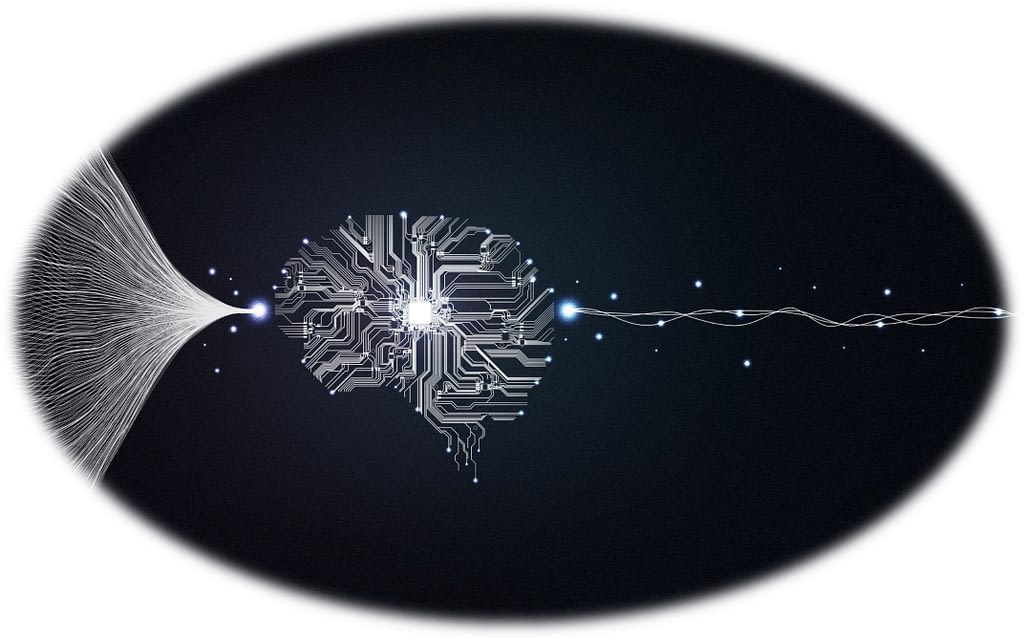
Strategic Use of Algorithms
The strategic use of algorithms allows businesses to manage vast amounts of data more efficiently and make decisions at a scale and speed that is not possible for human beings alone. This is particularly crucial in today’s fast-paced business environment, where being data-driven is key to staying competitive. (Brown, 2021)
However, it is essential for businesses to continuously update and fine-tune their algorithms to stay relevant and effective. The landscape of digital business is ever-changing, and algorithms need to evolve to keep up with new trends and technologies. Moreover, the abundance and quality of data are crucial in the effectiveness of algorithms. A rich dataset ensures that algorithms have enough varied information to learn from and make accurate predictions or decisions.
Garbage In, Garbage Out
Similarly, the quality of data is vital; high-quality, reliable, and relevant data helps in developing algorithms that are precise and effective in their tasks. Poor quality or biased data can lead to inaccurate results, making the role of data quality and abundance fundamental in the realm of algorithmic decision-making. GIGO stands for “Garbage In, Garbage Out.” It’s a principle in computing and data analysis that emphasizes the quality of output is determined by the quality of the input. If flawed, inaccurate, or poor-quality data is fed into a process or system, the results will also be flawed, inaccurate, or of poor quality. It underscores the importance of ensuring high-quality, relevant data in any data-driven operation or decision-making process.
“Without data you’re just another person with an opinion.”
W. Edwards Deming
Summary Conclusion
In conclusion, while algorithms may not entirely run a company, they play a critical role in various aspects of business operations, driving efficiency, innovation, and strategic decision-making. Their influence spans across different sectors, making them an indispensable component of modern business success.
Ready to transform your business with security by design? Contact Citanex today!
Related Articles
Bibliography
Statista, 2024. Volume of data/information created, captured, copied, and consumed worldwide from 2010 to 2020, with forecasts from 2021 to 2025. https://www.statista.com/statistics/871513/worldwide-data-created/
Brown, M. (2021, 10 06). Why Smart Algorithms Are The Future Of Business Success And Making Money. Retrieved from jarvee.com/: https://jarvee.com/why-smart-algorithms-are-the-future-of-business-success-and-making-money/
Ismail, S. (2024, 01 05). Why Algorithms Are The Future Of Business Success. Retrieved from growthinstitute.com: https://blog.growthinstitute.com/exo/algorithms
Joshi, N. (2016, 08 22). Retrieved from 4 industries that use the algorithmic business model successfully: https://www.linkedin.com/pulse/4-industries-use-algorithmic-business-model-naveen-joshi
Salesforce. (2020, 11 09). How Algorithms Actually Impact Your Business . Retrieved from salesforce.com: https://www.salesforce.com/ca/blog/2020/11/how-algorithms-actually-impact-your-business.html
Footnotes
- A zettabyte is a unit of digital information storage that equals approximately one sextillion (1,000,000,000,000,000,000,000) bytes or, more precisely, 270 bytes. This enormous amount of data is equivalent to a billion terabytes (TB) or a million petabytes (PB). Zettabytes are used to measure extremely large volumes of data, often in the context of global internet traffic, digital data storage, and the capacity required to handle big data analytics and the vast data generated by connected devices in the era of the Internet of Things (IoT). ↩︎
- Raymond Kurzweil is an influential American inventor, futurist, and author, celebrated for pioneering advancements in technologies like optical character recognition, text-to-speech synthesis, and electronic keyboards. Born in 1948, Kurzweil has made significant contributions to artificial intelligence and technology development. He is renowned for his theory of the Singularity, predicting a future where AI surpasses human intelligence by 2045, leading to profound changes in society. Kurzweil’s work has earned him the National Medal of Technology and Innovation and a place in the National Inventors Hall of Fame. He has also worked at Google, focusing on machine learning and language processing projects, and has authored books that explore the intersections of technology, health, and the future of human intelligence. ↩︎
- Paul R. Ehrlich is an American biologist and researcher best known for his work in the field of population studies and environmental issues. While he is primarily recognized for his warnings about overpopulation and its impact on Earth’s resources through his 1968 book, “The Population Bomb,” Ehrlich has also touched upon the limitations of the human brain in the context of understanding and solving complex environmental and societal challenges. His work suggests that human cognitive and perceptual limitations can hinder our ability to fully grasp the scale of environmental degradation and to implement the necessary solutions for sustainable living, highlighting the need for interdisciplinary approaches and innovative thinking to address global challenges. ↩︎
- Machine Learning is a field of artificial intelligence that enables computers to learn from data and improve their performance over time without being explicitly programmed for every task. By analyzing patterns in data, machines can make predictions or decisions, adapting their behavior to perform a wide range of tasks more effectively. Think of machine learning like teaching a toddler to sort blocks by color. Just as you’d show the toddler examples of red blocks in one pile and blue in another, machine learning teaches computers to recognize patterns by feeding them examples. Over time, just as the toddler learns to sort the blocks on their own, the computer learns to make decisions based on the patterns it has seen. ↩︎
- Deep Learning is a subset of machine learning that uses complex algorithms to model and process data in layers, mimicking the way human brains operate to learn from large amounts of information. Imagine deep learning as an intricate factory assembly line, where raw materials (data) enter one end, and as they move down the line, each station (layer in the neural network) processes the materials in increasingly sophisticated ways. By the time the materials reach the end of the line, they’ve been transformed into a finished product (a decision or prediction), with each station adding its own specialized touch based on the work of the previous ones, much like how our brain layers build on each other to process information and learn. ↩︎
- Predictive maintenance is a technique used to predict when equipment will fail or require maintenance, allowing for intervention before failure occurs, thus reducing downtime and maintenance costs. It uses data analysis tools and techniques to detect anomalies and predict equipment failures. ↩︎
Recent Articles
- COMPLACENCY: The Silent Threat – Why It Kills and Why It Matters
- International Intelligence Alliances: Threats to Data Privacy Unveiled
- Iran’s Cyber Warfare: Strategies and Global Defense
- Empowering the Future: Celebrating Women’s Triumphs and Tackling Challenges in Tech
- Beyond Human Limits: Can Algorithms Run a Company?